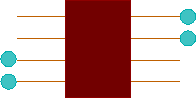
Network and Virtual Environments Group (in Spanish GREV) focuses on distributed virtual environments, interconnection networks and high performance computing. These systems are transversal to different kind of applications, but we have applied them to urban mobility, interconnected videogames or computational genetics. Since its establishment, the GREV group has carried out research in constant collaboration with the Conslider ACCA team. Last years the GREV has started interdisciplinary collaborations with research teams coming from computational genomics and augmented reality.
Scientific objectives
Research in the areas of computer architecture , computer science and artificial intelligence
Research lines:
- Asset location and tracking systemsin non-controlled environments. This line tries to improve real-time location and tracking systems for assets (people or goods) in industrial or construction sites, which contain any king of noise and changing conditions.
- Development of deep neural networks for new wavelet transforms. This line studies the combination of Deep Learning techniques with wavelet transformed data, applying this strategy to two different problems. First, the automatic analysis of human DNA methylation data, to obtain differentially methylated regions (DMRs). Second, the analysis and automatic detection of patterns in medical images.
- Distributed video coding/transcoding in the cloud. We focus on the development and improving of distributed architectures in the cloud for parallel coding and transcoding of the new video standards (AV1, VVC, etc.).
Líneas de investigación:
- Localización y seguimiento de activos en entornos no controlados. Esta línea trata de desarrolllar y/o mejorar soluciones de localización y seguimiento en tiempo real de activos (personas u objetivos) en entornos no controlados (principalmente entornos industriales con todo tipo de ruido y condiciones cambiantes).
- Desarrollo de redes neuronales profundas para nuevas transformadas wavelets. Estudia la combinación de deep learning con datos transformados wavelets, aplicando esta estrategia a dos tipos de problemas diferentes. Primero, el análisis automático (en la detección de DMR, sobre todo) de datos de la metilación de genoma humano. Segundo, para el análisis y detección automática de patrones en imágenes médicas.
- Codificación/Transcodificación distribuida de vídeo en la nube. Esta línea se centra en el desarrollo y mejora de las arquitecturas distribuidas en la nube para la codificación y transcodificación paralela de los nuevos estándares de vídeo (AV1, VVC, etc.).
- Soluciones de despliegue y configuración de servicios en el Edge, para la propuesta y evaluación de herramientas para el despliegue de servicios en dispositivos con bajo coste, bajo consumo y recursos limitados, como los empleados en el Internet de las cosas (IoT).